In the ever-evolving landscape of IT, Artificial Intelligence for IT Operations (AIOps) has emerged as a transformative force. Recent years have witnessed remarkable strides in AIOps, where vendors have introduced diverse strategies for event correlation, classification, and predictive analysis. Nevertheless, many of these methodologies still rely heavily on conventional AI techniques that demand extensive rule sets. This overreliance on rules presents a formidable obstacle to realizing AI’s true potential in IT operations. This article embarks on dissecting these common approaches and their inherent limitations. Moreover, it proposes a groundbreaking paradigm shift that promises to obliterate these constraints and usher in a new era of scalable value for organizations.
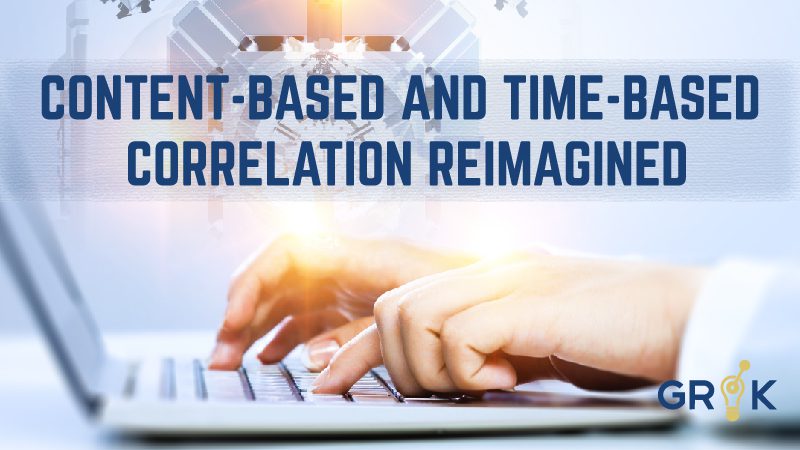
Content-Based and Time-Based AIOps Event Correlation Reimagined
In contrast to these traditional methodologies, content-based and time-based correlation approaches often operate outside Machine Learning (ML), relying heavily on static rule sets. Content-based correlation depends on predefined rules to categorize events, while time-based correlation groups events based on specific time intervals. However, These simplistic approaches struggle with complex environments. They often lead to multiple event clusters, which may relate to a single underlying issue
Consider, for instance, a network monitoring system overseeing a company’s IT infrastructure employing Content-Based Correlation. Here, events are grouped based on specific error codes. When a server encounters a “Server Down” error (error code: 503), all corresponding events are bundled together. However, if the same server experiences a “Database Connection Error” (also error code: 503), Content-Based Correlation might not differentiate between these two distinct issues, resulting in inaccurate event grouping.
Similarly, Time-Based Correlation clusters events occurring within predefined time windows, such as 10 minutes. While this approach may capture multiple server errors reported within the same timeframe, it might overlook the root causes of these issues. Unrelated events could be grouped within the same window, making pinpointing and addressing the underlying problems challenging.
Elevating Correlation with Text Semantic Clustering
Text semantic clustering injects a more profound AI sophistication into event correlation, albeit with potential room for optimization. Vendors often use text similarity and time windows for event clustering. However, this method may still need additional static rules for optimal performance. Consequently, it occasionally needs to improve in correlating events with diverse text summaries that are, moreover, linked to the same core issue. This can result in the formation of redundant and fragmented event groups.
Take, for example, a cloud service provider managing multiple data centers employing Text Semantic Clustering. This technique groups events based on their textual descriptions. The system identifies correlations if servers in various data centers encounter similar issues quickly. For instance:
- Server 1: “High CPU Usage on Server A.”
- Server 2: “Server A Experiencing Memory Overload.”
- Server 3: “Server A Disk Space Almost Full.”
Text Semantic Clustering recognizes correlations among these events, signaling a potential underlying issue affecting Server A’s performance. However, it may occasionally miss correlations between similar issues occurring in different data centers, necessitating the addition of static rules for manual grouping.
Exploring the Boundaries with Overlaying Time-Based Aspects
Including a time-based aspect alongside semantic clustering introduces an extra layer of complexity that may inadvertently limit correlation capabilities in vast and intricate IT and Network environments. Furthermore, as issues can span extended durations in such settings, rigid time-based rules may lead to many disjointed event clusters, hampering the resolution of overarching problems.
Topology Correlation: A Glimpse into Interconnectivity
In an ideal setting, topology correlation hinges on accurately representing the intricate interconnections between Configuration Items (CIs). Regrettably, maintaining a fully precise Configuration Management Database (CMDB) or topology discovery engine is often a formidable challenge for organizations. As a result, this difficulty in maintaining accuracy can hinder the successful implementation of topology-based correlation approaches.
Anomaly Detection on Log Frequency: A Supplementary Perspective
While effective in detecting unusual log behaviors, anomaly detection based on log frequency typically provides a supplementary view of potential issues within logs. However, it must possess the comprehensive correlation capabilities required for holistic incident resolution or event volume reduction, making it a valuable but limited tool in the arsenal of AIOps.
We’ll explore these correlation methods in the next sections, examining their strengths, weaknesses, and real-world uses. This will illustrate how AIOps can revolutionize event correlation for organizations.
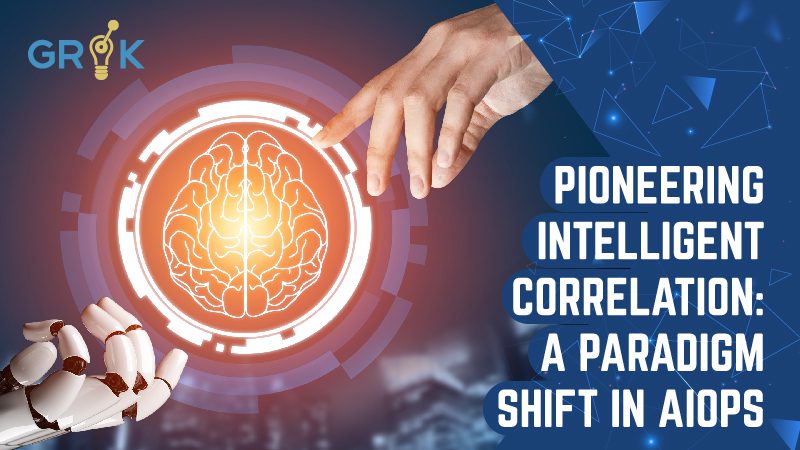
Pioneering Intelligent AIOps Event Correlation: A Paradigm Shift in AIOps
In the quest to transcend the limitations that have long constrained traditional AIOps event correlation, we advocate a transformative paradigm shift towards intelligent correlation. This shift entails embracing cutting-edge Machine Learning (ML) algorithms and techniques, breaking free from the shackles of static rules. At its core, this visionary approach is underpinned by several pivotal components:
Empowering Event Clustering with ML:
- Embracing sophisticated ML algorithms for event clustering revolutionizes how related events are grouped. This dynamic approach liberates us from predefined rules and empowers ML models to discern real-time patterns and associations. The outcome is a more precise and flexible correlation across multiple dimensions, unburdened by the rigidity of traditional methods.
The Dawn of Adaptive Time Windowing:
- The integration of adaptive time windowing techniques ushers in a new era where correlation adapts to the specific nature of each issue. These techniques allow the system to flexibly adjust time boundaries, ensuring that correlations encompass extended periods. This adaptive flexibility equips organizations with the tools for more comprehensive and effective problem resolution.
Elevating Classification with Machine Learning:
- One often overlooked aspect in traditional AIOps is the power of Machine Learning-based classification. Instead of relying on static rules to define event groupings and their association with incidents or problems, the ML-based type dynamically assigns incidents or issues to event clusters. This dynamic approach enhances accuracy, reduces manual rule management, and ensures a more agile response to emerging issues.
The path to achieving scalable and highly effective AIOps event correlation necessitates a departure from the confines of rule-based methodologies. The future lies in intelligent correlation, fortified by advanced ML algorithms, contextual awareness, adaptive time windowing, and ML-driven classification. This approach promises significant value and a streamlined and agile incident management process by liberating organizations from the burdensome task of extensive manual rule creation and maintenance. It’s a revolution poised to unlock AI’s full potential in the dynamic landscapes of IT and Network environments, setting the stage for a brighter and more efficient future in AIOps.